Adaptive Learning: A Stabilizing Influence
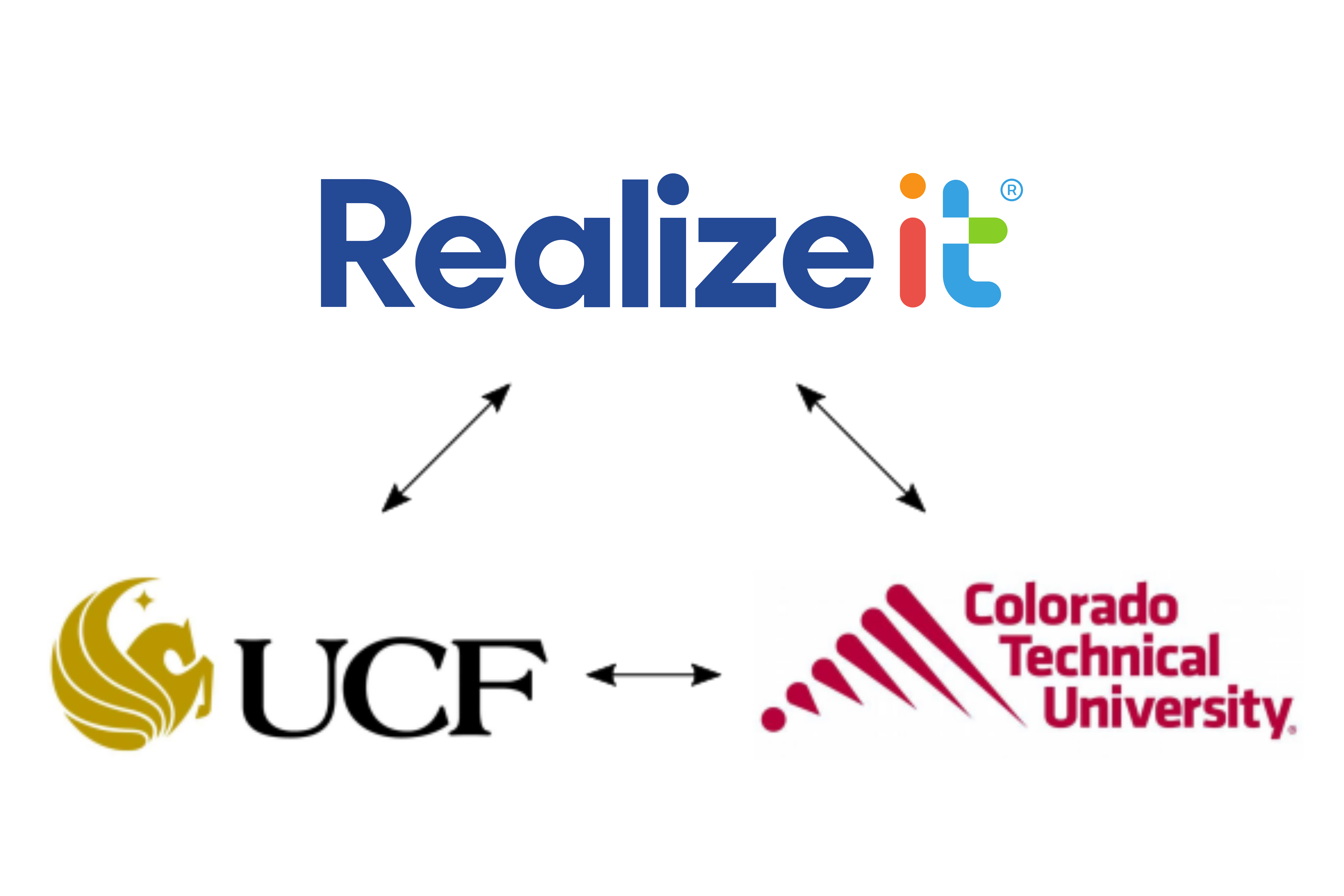
Recently we had a paper Adaptive Learning: A Stabilizing Influence Across Disciplines and Universities published in the Online Learning Journal based on some of the collaborative research that we have been doing with two partner institutions, the University of Central Florida (UCF) and Colorado Technical University. Before I get on to talking about the paper itself, I want to tell you a bit about this partnership as we see this as a great model for how we would like to work with others.
An Adaptive Partnership
Both UCF and CTU are early adopters of adaptive learning. We began working with CTU in 2012 and UCF in 2014. These are two very different institutions in how they are set up, how they use adaptive learning and the population of students they serve. UCF is a research-intensive university, and from their very first trials of Realizeit, we have been working with Chuck Dziuban and Patsy Moskal from the UCF Center for Distributed Learning to understand the impact of adaptive learning on students and learning. The formation of a collaborative relationship between all three organizations occurred organically as people from UCF and CTU began to meet at conferences and learn of each other’s work. Connie Johnson, CTU’s chief academic officer and provost, has led their effort to share their experiences and success with adaptive learning.
What makes this collaboration so successful, and why we see it as a great model for collaborating with others, is that each of us brings something different to the relationship. Not only do we get different perspectives on adaptive learning from the differences between the institutions, but each organization also bring different capabilities. UCF brings their considerable research experience and expertise, CTU has successfully taken adaptive to scale, and Realizeit brings expert knowledge of the system and the data it produces. Together we have produced research ranging from students’ perceptions of adaptive learning to how stable the underlying structure of adaptive learning is across domains and institutions. You can see the papers we have produced on our Papers page.
A Stabilizing Influence
In our most recent collaborative work, we sought to examine and understand the hidden dimensions underlying the adaptive learning process. Combining the data from both UCF and CTU would allow us to make comparisons across multiple disciplines and in two very different universities. Our objective was to determine if differing disciplines and university contexts alter the learning patterns, thereby impacting on effectiveness. This has implications for the fields of learning science and predictive analytics. If the underlying dimensions vary considerably by context, then it creates difficulties for how we translate what we’ve learned, and the models of learning that we build, from one context to another. I’m only going to give a high-level overview here of our work and findings but be sure to check out the full paper for more details.
The primary technique used in this work is Principal Component Analysis (PCA). While you don’t need to have expert knowledge of this technique to understand the paper, knowing some of the basics would help, so I’ve provided a quick introduction in this post.
The Courses and the Data
For this paper, we concentrated on courses in mathematics and nursing. In the table below, you can see the list of courses chosen and the number of students and sections in each course.
Institution | Course | Students (Sections) |
---|---|---|
UCF | Intermediate Algebra | 332 (2) |
College Algebra | 363 (5) | |
Pathophysiology | 537 (9) | |
CTU | Introduction to Algebra | 6,693 (38) |
Analytic College Algebra | 4,486 (26) | |
Trends in Contemporary Nursing | 303 (30) |
For the study, we used 13 variables generated by the Realizeit platform. These describe the students’ outcomes and engagement.
The Analysis
We found these to be intercorrelated, so we subjected them to the PCA procedure. We performed this for each of the individual courses in each institution, and for the combined sample of all three courses in each institution. This generated a total of eight pattern matrices, one for each sample. We retained components with an eigenvalue greater than one and used pattern coefficients with an absolute value greater than .40 to help form an interpretation of the components. I’ve included one of the pattern matrices below.
Component | ||||
---|---|---|---|---|
C1 | C2 | C3 | C4 | |
Calculated | 0.95 | |||
Knowledge covered | 0.95 | |||
Knowledge state | 0.91 | |||
Determine knowledge | 0.79 | |||
Average score | ||||
Total activities | 0.97 | |||
Num. revised | 0.90 | |||
Num. practiced | 0.61 | |||
Interactions | 0.98 | |||
Messages sent | 0.98 | |||
Knowledge covered growth | 0.93 | |||
Knowledge state growth | 0.92 | |||
Total time | 0.44 |
The pattern matrices capture the structure of adaptive learning in each of the samples. To answer our question on whether the organization of adaptive learning is constant or if the patterns change by institution or course context, we needed to compare the 8 pattern matrices (called component solutions in the paper) to each other.
In our work, we examined the 28 possible pairwise comparisons of the eight patterns. In the paper, we limited the comparison to the 10 comparisons given below, but the results hold across all possible comparisons.
- Internal Institutional Comparisons – comparing patterns across samples within an institution
- UCF (3 samples → 3 comparisons)
- CTU (3 samples → 3 comparisons)
- Cross-Institutional Comparisons – comparing patterns from samples across institutions
- Entire Samples (2 samples → 1 comparison)
- Course Level
- Comparison of the matched algebra courses (2 sets of 2 samples → 2 comparisons)
- Comparison of the two nursing courses (2 samples → 1 comparison)
To make the comparisons, we used a metric known as the Tucker congruence coefficient. You can think of it as a correlation type metric for pattern matrices. We generated this metric for each component pair in each comparison and each comparison as a whole. There is far more detail in the paper as we talk through each comparison, but in summary, we found that they all match. In the samples available to us, the organization of adaptive learning is constant across institution and course context - we found the same four components in each of the samples. But what are these components? How do we interpret them in real-world terms?
The Components
By examining how the four components relate to each of the original variables we derived the following interpretation. (Note in some patterns C3 and C4 swap position.)
- C1: Knowledge Acquisition relates to achievement and has a mastery element associated with it. Knowledge acquisition in adaptive learning assesses learning before, during, and upon completion of a course and forms the benchmark for student success. Also, it serves as the basis of the decision engine’s recommendation about the appropriate learning path for students and an early indication of possible difficulties in the learning sequence.
- C2: Engagement Activities bears a strong relationship to what Carroll called the time students spend in actual learning and relates to how much energy a student expends in the learning process. If one could hold ability level constant, a reasonable assumption might be that students who are more engaged in learning activities will score higher on knowledge acquisition.
- C3: Communication emerges in the Realizeit platform, enabled by messages sent and interactions. This is the social dimension of adaptive learning and the way students communicate with each other and their instructors. At another level this component underlies the effort expended communicating in courses.
- C4: Growth is a clear expectation for any course. Measuring change in knowledge acquisition can result from many baseline measures and is an essential element of the learning cycle. Growth is the change in what information a student has mastered and is the critical bellwether for student progress in their adaptive learning courses.
As stated in the paper, these four components should be no surprise, because educators know that this underlying pattern is fundamental to effective teaching and learning in all modalities, not just adaptive.
Conclusion
So what next? These components provide us with a solid basis on which to stand and to begin to make comparisons across disciplines and institutions. Instead of comparing individual metrics, we can now talk about student performance on constructs which we know are fundamental across learning. Indeed this is our next step. We should be sharing some of this work soon, so make sure to check back.
As always if you have any questions and comments, or want to get involved in any of the research then just let us know.
Reference for this paper
Dziuban, C., Howlin, C., Moskal, P., Johnson, C., Parker, L., & Campbell, M. (2018). Adaptive Learning: A Stabilizing Influence Across Disciplines and Universities. Online Learning, 22(3). doi: http://dx.doi.org/10.24059/olj.v22i3.1465