Welcome to Realizeit labs
This site is where we'll share some of the inner workings of the Realizeit Research and Development team: the projects we're working on, meetings we're attending, collaborations we're involved in, papers we're publishing. Make sure to follow us to stay up to date on what is happening.
If you are interested in using the Realizeit platform, please visit www.realizeitlearning.com.
We are always interested in hearing new ideas and discussing potential research collaborations. If you are interested in working with us, or one of our partner institutions on a research project then let us know.
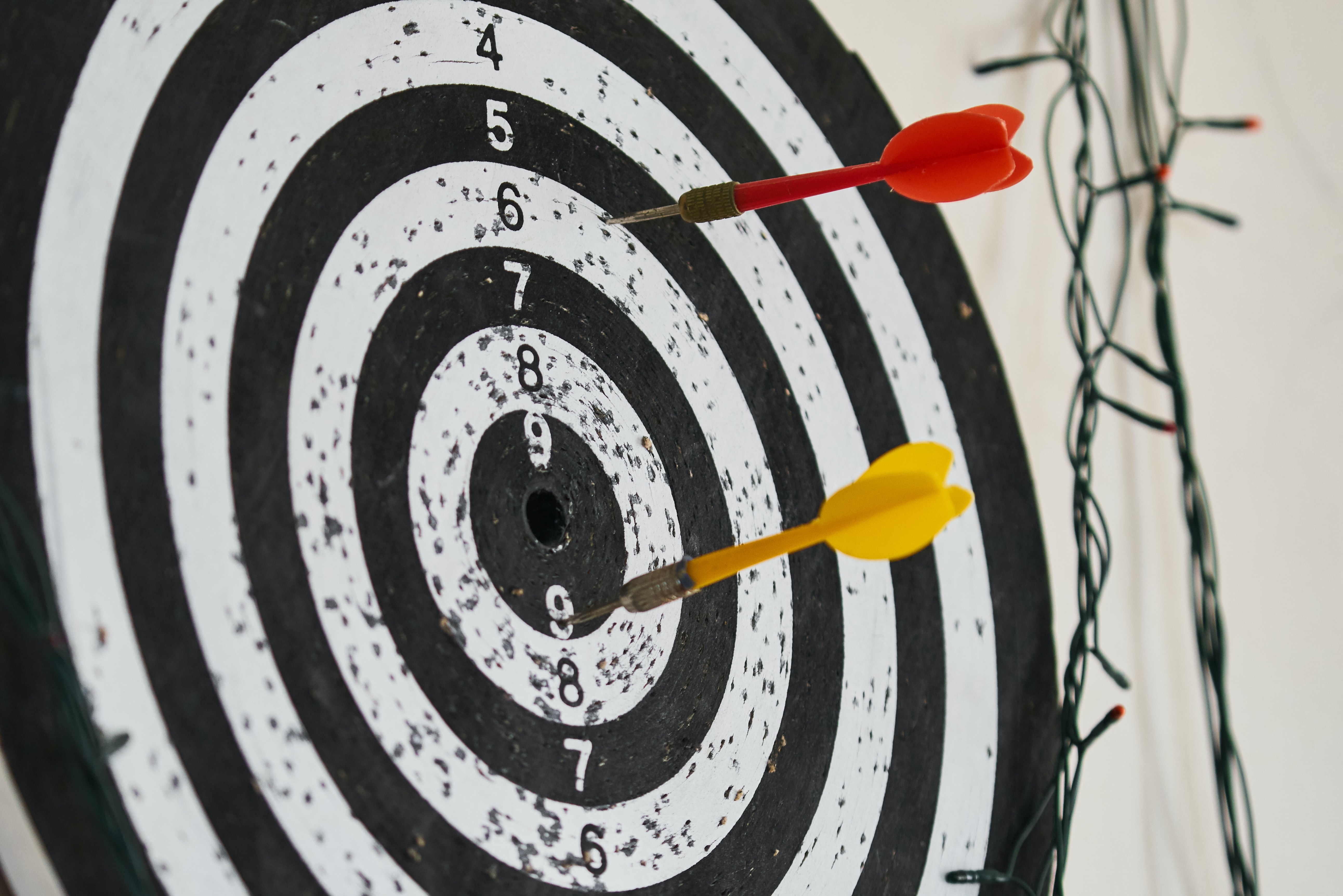
Adaptive Analytics: Predictive Modeling Based on Teachable Skills at UCF
Jan 4, 2021 • Colm Howlin
We have recently collaborated with the University of Central Florida and Colorado Technical University on building models that can predict student success at the course level for College Algebra. The...
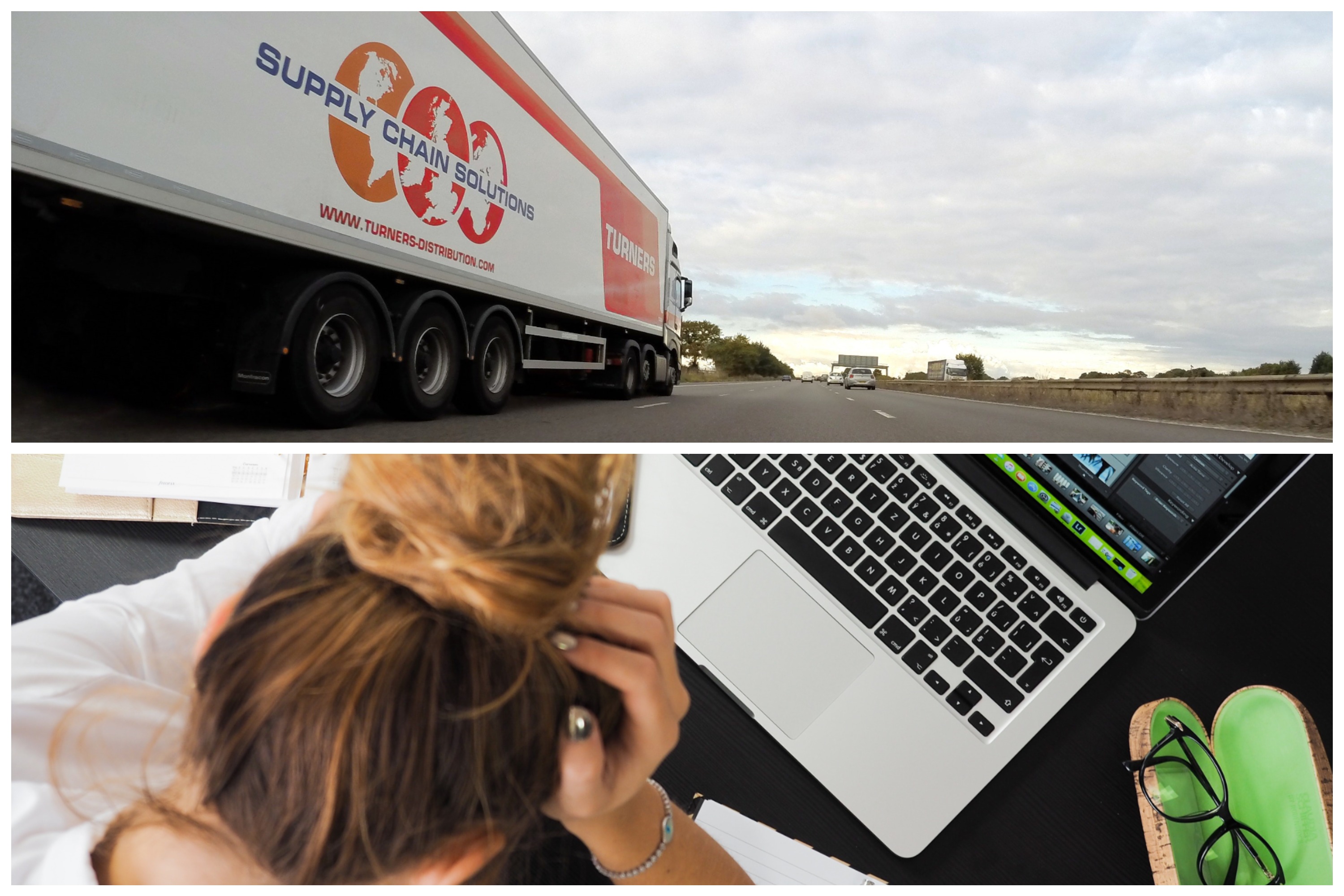
Supply Chain Management and Adaptive Learning
Jan 26, 2020 • Andrew Johnson, Chuck Dziuban, Mitchell Eid & Colm Howlin
There is an adage that there is nothing new under the sun. However, often, dissimilar terminology, contexts and time frames obscure commonalities in theories and problem solutions. For example, Steven...
A preclinical hybrid curriculum and its impact on dental student learning outcomes
Journal of Dental Education 2020; 1-11. doi:10.1002/jdd.12517
View abstract Go to paper
Abstract
Objectives
The purpose of this study is to measure how the implementation of an online, preclinical hybrid curriculum impacts dental student clinic readiness, the outcomes of grades, critical thinking skills, and student and faculty perceptions respectively.
Methods
This longitudinal comparative and descriptive study used objective data and subjective (survey) data for 4 dental class cohorts. Groups A and B experienced a traditional lecture‐based curriculum, while Groups C and D experienced a hybrid curriculum that was lecture‐free and implemented active learning. The Health Sciences Reasoning Test (HSRT), an objective assessment, was used to measure students’ critical thinking skills.
Results
Dental student outcomes have either remained steady or improved with the transition to a new hybrid curriculum. According to the student and faculty survey results, the hybrid curriculum promoted student learning, independence, critical thinking, initiative and self‐motivation, and clinic practice readiness. Group C (N = 68) Total Online Platform mean scores demonstrated a significant and moderately strong correlation with the preclinical course mean grades (r = 0.68, P = 0.00). Group D HSRT (n = 63) for Attempt 1 (end of year 1) and Attempt 2 (end of Year 2) paired T test resulted in HSRT Overall (mean difference = −2.27, SD = 7.21, t = −2.5, P = 0.02) for the second preclinical year.
Conclusion
The hybrid curricular approach afforded many benefits. Faculty took an active role in imparting knowledge when compared to the lecture hall. Having students immersed in continual assessment through an online adaptive platform and active learning promoted self‐motivation, deeper learning, applied knowledge, and discouraged superficial memorization.
Adaptive Analytics: It’s About Time
Current Issues in Emerging eLearning: Vol. 7 : Iss. 1 , Article 4
View abstract Go to paper
Abstract
This article describes a cooperative research partnership among a large public university, a for-profit private institution and their common adaptive learning platform provider. The focus of this work explored adaptive analytics that uses data the investigators describe as metaphorical “digital learning dust” produced by the platform as a matter of course. The information configured itself into acquired knowledge, growth, baseline status and engagement. Two complimentary models evolved. The first, in the public university, captured end-of-course data for predicting success. The second approach, in the private university, formed the basis of a dynamic real-time data analytic algorithm. In both cases the variables that best predicted students at risk (effective use of time and revision attempts) were deemed teachable skills that can improve with intervention.
Detecting Outlier Behaviors in Student Progress Trajectories Using a Repeated Fuzzy Clustering Approach
Proceedings of the 12th International Conference on Educational Data Mining, 2019
View abstract Go to paper View slides
Abstract
Clustering of educational data allows similar students to be grouped, in either crisp or fuzzy sets, based on their similarities. Standard approaches are well suited to identifying common student behaviors; however, by design, they put much less emphasis on less common behaviors or outliers. The approach presented in this paper employs fuzzing clustering in the identification of these outlier behaviors. The algorithm is an iterative one, where clustering is applied, outliers identified, the data restricted to the outliers, and the process repeated. This approach produces a clustering that is crisp between each iteration and fuzzy within. It arose as a consequence of trying to cluster student progress trajectories in an adaptive learning platform. Included are results from applying the repeated fuzzy clustering algorithm to data from multiple courses and semesters at the University of Central Florida, (N=5,044).